In the last few years, it has become technically and economically feasible to capture and store huge quantities of data. Businesses generate a vast amount – but the reality is, few know what to do with it. The IDC estimates that only 0.5% of data is ever analysed, which considering the potential benefits, is a remarkable statistic. Competitors in business data analytics are cornering the market; just look at classic examples like Amazon and Netflix.
At a moment when many companies offer comparable products supported by similar technologies, business processes are arguably the last bastion of differentiation. Companies that can predict what their customers want, what price they’re willing to pay, how often they’ll return, and what leads them down the sales funnel will inevitably have the edge. This also functions internally; true analytic competitors calculate how personnel contribute or detract from revenue. They can also closely track inventories, predict demand patterns, and achieve a high rate of perfect order fulfillment.
Here, we’ll look at the route to analytic competitiveness and the processes and people that support its progression through the various stages, as outlined in ‘Competing on Analytics: The New Science of Winning’, Thomas Davenport and Jeanne Harris’s seminal (and recently updated) report. With this framework, you can identify where your organisation sits on the scale and draw up a strategy relevant to your business data analytics goals.
Table of Contents
Stage 1: Analytically impaired
Everyone has to start somewhere. The first stage of analytic development is when an organisation is analytically impaired. Essentially, this means the company is flying blind; they’re applying reactive strategies to customer interactions and operational capabilities, and they’re plagued by missing or poor quality data. Equally, systems will be disparate and poorly integrated, with poor definitions of data.
This, naturally, implies starting from scratch. Much of the time and money should go into technological tasks: refining transaction systems, making data available in warehouses, selecting and implementing analytic software, and assembling the IT environment. However, tools are nothing without implementers and operators. Organisations will need to source the personnel they need to make it happen. For instance, when Harvard Business School veteran Gary Loveman moved from academia to the corporate sphere at Harrah’s, the first thing he did was draft in statistical experts. These experts were hired to design a marketing data analytics programme that took a quantitative approach to customer loyalty.
Existing employees will also need to become better acquainted with advanced analytics. They need to be aware of what data are available and how it can be analysed. They also need to know how to identify ‘bad data’: data that are incomplete, duplicated, or poor quality. Equally, they need to play a key role in amassing data, as generating a usable data lake is an ongoing project. For example, verifiable trends may only emerge in the latter half of a years-long initiative.
Stage 2: Localised analytics
Once the foundation is laid, businesses can begin to execute specific analytics projects. Generally, these projects are isolated and relatively limited in scope; but this isn’t to say that this isn’t an important stage in expanding business intelligence and analytics across the organisation. Although these projects generally won’t aid high-level decision-making, they’re a step in the right direction.
Generally, a good strategy is to pick several functions that together, contribute to an overarching business goal. To reflect again on the Gary Loveman example, energy was channelled towards increasing customer loyalty, and subsequently, related areas like pricing and promotions. The key here is to not get too embroiled in the details of one function-specific analytics project – always maintain focus on the overarching goal to graduate onto the next stage of analytic competitiveness.
This links to the allocation of resources and how applicable certain functions are to big data technologies or predictive analytics. However, the possibilities are expanding – previously ‘unquantifiable’ metrics like customer behavior and brand perception can now be tracked. For instance, the advertising industry is embracing ‘econometrics’, which is a statistical data analysis technique for quantifying the lift provided by different promotions over time.
Stage 3: Analytical aspirations
Before his retirement from food giant the Sara Lee Bakery Group, former CEO Barry Beracha kept a sign on his desk that summed up this analytic competitor’s philosophy: “In God we trust. All others bring data.” This neatly summarises the third stage of analytical maturity: when executives make a commitment to the wider use of analytics. However, they need backing.
Thus, this stage is characterised by a proliferation of business data analytics tools and a growing data lake, but a gap in know-how when it comes to dealing with unintegrated or unstructured data. In a study conducted by the Harvard Business Review, they commented that in many companies where an individual business leader was trying to advocate for advanced analytics, lower-level staff lacked the perspective to get on board.
CEOs and employees alike are likely to need help grasping business data analytics for the principles to permeate corporate culture. Businesses should turn to experts to push for analytical strategies throughout the organisation, translating technical terms into plain language. With the right people, with the right blend of hard and soft skills, this ethos can begin to spread throughout every echelon of the organisation.
Stage 4: Analytical companies
A company has reached analytic maturity when business data analytics is an enterprise-wide capability. Data in business is seen as a corporate priority, and subsequently, the organisation has an overarching analytic strategy backed by high quality data. This plan will include IT processes and governance principles, and an outline for some automation.
This is supported by a doubling down on analytic culture. Employees must have a healthy respect for measuring, testing, and appraising quantitative evidence; every decision must be based on cold, hard facts. Management should support this culture by demonstrating a commitment to analytics. To use the example of Sara Lee Bakery Group once more, ex-CEO Beracha was nicknamed the ‘data dog’ by his employees as he hounded them for data to support every decision.
There are, of course, scenarios within which a decision to optimise a process or implement a new project needs to be made fast. This often means there won’t be time for extensive analysis. For instance, Jeff Bezos is known to prefer rigorous quantification of customer behavior before rolling out new features. However, he couldn’t test the retailers’ ‘look inside’ feature for book sales without applying it to enough inventory. It was also expensive to implement, which increased the risk. But Bezos trusted his instincts and the feature was a success. This demonstrates what you need – an analytical, informed foundation, with the flexibility to move with agility.
Stage 5: Analytical competitors
Any organisation can execute a basic statistical data analysis, but the mature iteration of data science for business will go well beyond the numbers. They will use predictive analytics to identify high-value customers, model retention rates, and develop loyalty programmes according to these metrics. They’ll pool data generated in-house with external sources for a complete picture of their customers’ demands and expectations. They’ll optimise their supply chains and predict setbacks, simulate possible solutions, and rapidly implement these responses. They’ll set price points in real-time to maximise conversions and create detailed models of how costs relate to performance.
Analytic competitors also run complex simulations to measure the impact of their strategies. They’ll then apply these results to continuously improve; for instance, credit card provider Capital One runs over 30,000 simulations a year, ranging from interest rates to customer incentives, among other variables. The goal is to entice customers, while ensuring that they’ll pay back their debts.
Progressive insurers employ a similar strategy by analysing granular customer data according to demographics. This will be extremely specific; for instance, they’ll be a distinct model for motorcycle owners over 30 with university degrees, a clean license, and a certain credit score. They’ll perform a regression analysis to identify the losses that this group accounts for, simulating the financial implications of insuring this particular customer profile. This will influence price setting, enabling the insurer to maintain profitability across a range of demographics.
This allows them to capture more business, where traditional insurers would reject a customer flat out. Herein lies the core of mature business data analytics – a remarkable level of nuance that enables businesses to make evidence-based decisions about previously intangible variables and influences.
The right technology is nothing without the right people in business data analytics
The key to analytic maturity boils down to one essential principle: analytic organisations hire analytical people. The real competitors hire the best and only the best will do. Capital One, who we mentioned above, employed what could only be described as an elite squad of data scientists to carry out simulations, and with the results, design products and offers.
Projects such as these call for a specialised skill set, including high-level problem solving ability, experience in project management and process improvement tools, as well as the required hard data science skills. Sure, many firms hire data scientists, but analytic competitors will hire them in large numbers. To reference Capital One once more, they hire three times as many data analysts as operations personnel. According to one executive, analysts are the primary role within the organisation – which generally speaking, is quite unusual for a bank.
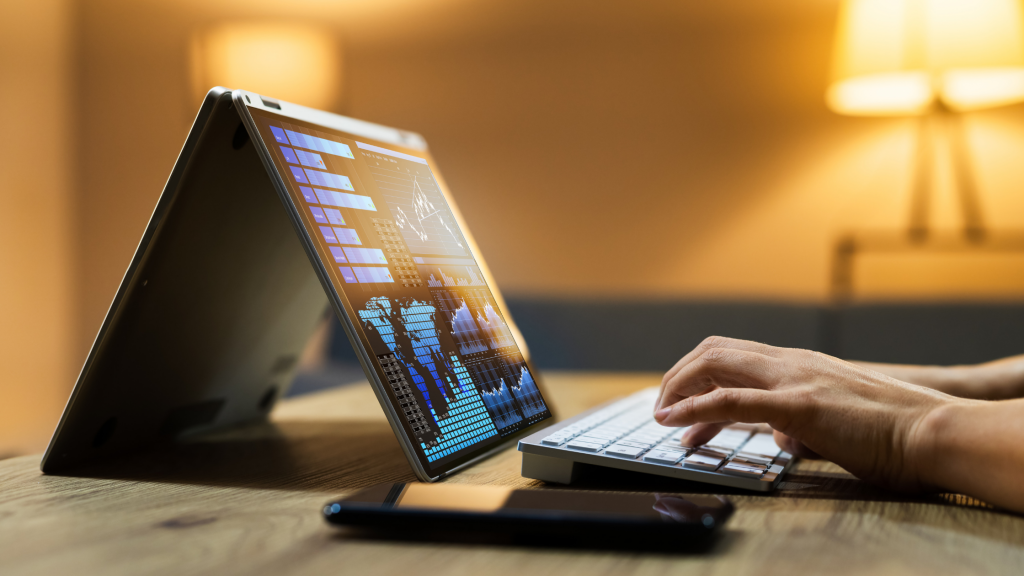
However – as touched on in the stage 5 description above – a good analyst needs to go beyond the numbers. An exceptional data scientist will have the soft skills to explain complex ideas in simple terms and have the people skills to interact with key stakeholders. The analyst needs to have a seat at the table with key decision makers, and they need to be able to hold their own, which is essential to organisational buy-in at every level.
This unique combination of analytical, business and people skills is a challenge to find. Certainly, an expert in SAS analytics or recursive partitioning is a particular personality type – and finding this breed of expert who also has the charisma to persuade decision makers can seem a little like hunting a unicorn. For some firms, recruitment processes can take over a year, with exhaustive head hunting and interview processes that are resource intensive unto themselves.
Oftentimes – and unfortunately – local labour markets aren’t exactly overflowing with this exceptional talent. Some innovative organisations are looking further afield to source the analytics talent that they need, drafting in remote experts to fill the gap. However, this can prove to be a barrier when firms need face-to-face interaction between experts and C-levels. Sure enough, the COVID-19 crisis has compelled us to all warm to digital interactions, but there is still a strong case for in-person negotiations.
Source the business data analytics expertise you need
Technology is important – but people are really what will drive a company towards analytical maturity, and indeed, competitiveness. At every stage of the journey towards becoming an analytic competitor, from the analytically impaired to data-driven enterprises, companies need specific experts. Whether it’s to build a data architecture or design complex scenario simulations, you need the right people at the right time.
This people power is what inspired the creation of Outvise, a unique talent pool of highly experienced TMD professionals. These professionals are sourced and certified by our dedicated team of head hunters, which recruit freelancers with this elusive combination of hard and soft skills. With specialisms ranging from data architecture, engineering, advanced analytics, project management, and consulting, organisations can source the talent they need more efficiently than ever.
Equally, Outvise understands the value of flexibility. Our professionals are available for on-site and remote projects – so depending on the nature of the initiative and the businesses specific needs, you can draft in on-site expertise or hire remote experts. For every stage and project scope, there’s valuable expertise at your fingertips. Explore the portfolio of exceptional digital, tech and business talent here.
Eusebi is Co-Founder and CEO at Outvise, with a demonstrated history in the management consulting industry. He's a seasoned entrepreneur with a strong background in Business Planning, Entrepreneurship, Strategic Partnerships, Business Transformation, and Strategic Consulting.
No comments yet
There are no comments on this post yet.