According to projections from management consultancy giants PwC, artificial intelligence (AI) will add a staggering $15.7 trillion to the global economy by 2030. Why? Because AI is the Swiss Army knife of technology; there are myriad applications to make businesses more effective, efficient, and ultimately, more profitable. This is the case across industries and sectors, from manufacturing to public services. So long as you have the data maturity, the possibilities are endless.
The most technologically advanced organisations have already applied artificial intelligence technology to remarkable effect. For example, a project at Mount Sinai medical group developed a machine learning programme to predict the development of cancerous cells with up to 94% accuracy. By aggregating historic patient and clinical data, doctors can make profoundly educated guesses about the progression of a variety of cancers.
Subsequently, businesses and public sector organisations alike are scrambling to get a slice of the pie. Currently, estimates suggest that the value of the AI industry will reach $118 billion by 2025. However – despite the incredible enthusiasm – almost 85% of all AI projects fail. This is why I developed my model for enterprise data maturity, which we’ll discuss here. Earlier iterations have been presented at the DeveloperWeek Europe 2021 conference and used as a part of data science workshops with Headfound GmbH, the Hyper Island digital school, and the University of Bundeswehr. Let’s look closer.
Table of Contents
Roadblocks to successful AI projects & data maturity
So what’s stopping these AI projects from working? As the old saying goes, where there’s a will there’s a way, so why are even the largest corporations losing their focus when it comes to AI and machine learning? Analysts suggest that there are three key barriers to AI adoption: enterprise maturity; fear of the unknown; and knowing where to begin. We’ll break this down.
Enterprise maturity consists of staff skills, data scope and quality, and governance issues. This is often the core of the failure of an AI project. Data is insufficient, poor quality and badly managed, and to add insult to injury, the company’s staff don’t have the skills to deal with it. This makes a problem go from bad to worse, as poorly trained staff make the data situation increasingly incomplete and chaotic.
Data illiteracy within an organisation also tends to breed distrust of the associated solutions. The second barrier, the fear of the unknown, is generally characterised by a lack of understanding of the benefits of AI. Leadership and staff alike are unsure of how to measure its value, and instead, focus on the risks. Frequently, these will be to do with security and privacy concerns, which in regions like Europe, are implicated in an increasingly rigorous regulatory environment.
But, even without fear of the unknown, some organisations just don’t know where to start. They know AI has benefits, but they don’t know where to apply it. This naivety often extends to the AI strategy in general, which can lead to AI-for-the-sake-of-AI projects, which in turn, create sub-par solutions. Other times, it might be simply because the company can’t find the funds to get the project going.
Certainly, these issues are complex, intertwined, and often, deeply rooted in company culture. However, there are active steps that can be taken to overcome these three obstacles and their substrata. The answer is data maturity.
The four dimensions of data maturity
To quote what’s becoming somewhat of a ubiquitous phrase in this day and age, data is the new oil. Data is the key to profitability, which is why its collection, cleaning, storage and analysis should be a priority for every enterprise. When this is done well, a company will reach data maturity, which, in short, is a company’s ability to create value with data. This, in turn, translates to successful AI projects.
So, if these are the characteristics of data maturity, how is it achieved? Essentially, this happens across four dimensions: strategy, technology, people and the data itself. ‘Strategy’ covers aims, use cases, and budgets and responsibilities. ‘Technology’ is about the tools in your arsenal and ‘people’ is about the team’s ability to use them. Finally, ‘data’ is the source, quality and architecture of the information the company holds.
Via the analysis of these four dimensions, companies can identify the areas where they fall short and take pointed, active steps to address these deficiencies. In my experience, a particular lack in one of these four dimensions results in four potential scenarios. These scenarios are often characterised by particular types of organisations and require specific action.
Scenario 1: Walking in the fog without an AI strategy
As the title suggests, this scenario is where a business lacks a coherent AI strategy. Usually, it’s big enterprises that are blighted by this issue. They aspire to develop their AI capabilities but don’t really know what to do except throw money at it. The tools are in place, but there’s no clear understanding of how to use them, never mind create value.
Key business questions:
- Which projects should be prioritised and how?
- How can we convince key stakeholders of the benefits?
- How can we develop a coherent strategy?
Basic action plan:
- Create and prioritise an AI use case portfolio.
- Implement a pilot project to demonstrate value.
Scenario 2: Master of none, or who’s going to build it?
However, a lack of data maturity isn’t a problem exclusive to corporate dinosaurs. Even small, seemingly nimble companies can fall short if they lack the people to complement their technological capabilities. Often, the IT department will be overwhelmed with requests that it can’t fulfil because they don’t have the data science expertise. They’ll hire consultants to fix the problem, but without the internal know-how to explain what’s going on, these external professionals are rendered all but useless.
Key business questions:
- Who do we hire to work with data and develop algorithms?
- How can we ensure data consistency and security?
- How do we encourage the adoption of solutions?
Basic action plan:
- Organise training about basic AI technology and operational implications.
- Hire a data scientist to lead a pilot project and train an internal data champion.
Scenario 3: But it works on my laptop!
Often, this is the retort of professionals from mid-size enterprises with unstructured, decentralised IT. Sure, this may seem like an agile model from a financial point of view, but when it comes to data maturity it can breed chaos. If there is even an IT department to speak of, it hasn’t got the technological scalability to handle an AI project. The projects are likely to start with one-time extracts built on local laptops that aren’t maintained or monitored. This renders productive implementation virtually impossible.
Key business questions:
- How are our business processes executed and tracked?
- What AI projects are possible to undertake?
Basic action plan:
- Create and prioritise an AI use case portfolio.
- Identify technology gaps and create a roadmap.
- Collect the right data for the execution of AI projects.
Scenario 4: The law of bad data, or garbage in, garbage out
Dirty data can happen to anyone. Generally, a lack of data maturity in regard to the data itself will happen to a company that’s just starting to focus on its data capabilities. It won’t have clear data governance guidelines and data availability, quality and quantity will be major roadblocks for AI projects. Generally, IT will be au fait with the systems in place but will struggle to identify the modules, tables, and columns that are relevant to the different functions.
Key business questions:
- Which processes and activities generate our data?
- What use cases can we build with our data?
- Who owns the data and who is responsible for its quality?
Basic action plan:
- Create and prioritise an AI use case portfolio.
- Define data responsibilities (owner, steward, etc.).
- Collect the right data for the execution of AI projects.
Defining an AI use case portfolio
So, you’ve done a data maturity assessment and identified areas where the organisation is lacking. So what now? Of course, the course of action will be unique to every enterprise. However, a theme that emerges in almost every scenario is the need to create an AI use case portfolio and prioritise these proposals. Before concluding, we’ll briefly reflect on how this is done. The process begins with an analysis of the value chain.
Primarily, you need to think about how AI could streamline every process, from procurement to manufacturing and development, right through to the sale and beyond. Let’s take lead nurturing as an example; what are the problems we have right now? How could artificial intelligence make this process more streamlined? Do we have the available data and relevant tech reqs? How would we act on this and what would be the desired result? You can apply this model to a variety of processes, from recruitment to order management and warehouse planning.
The next phase will be prioritising these potential use cases. This analysis can be divided between two main umbrellas: business impact and feasibility. When assessing business impact, you need to consider the value models, the potential for user adoption and the risks. When it comes to feasibility, you need to think about the duration and cost of the project and the availability of associated tools. This will often intertwine with the project’s dependency on other departments.
Often, a straightforward way to prioritise a use case portfolio is to create a diagram like the one shown below. With this visualisation, you can clearly identify the return on investment of a given tool. We’ve added some suggestions to give an idea of how different solutions generally operate in terms of impact and effort for any given organisation.
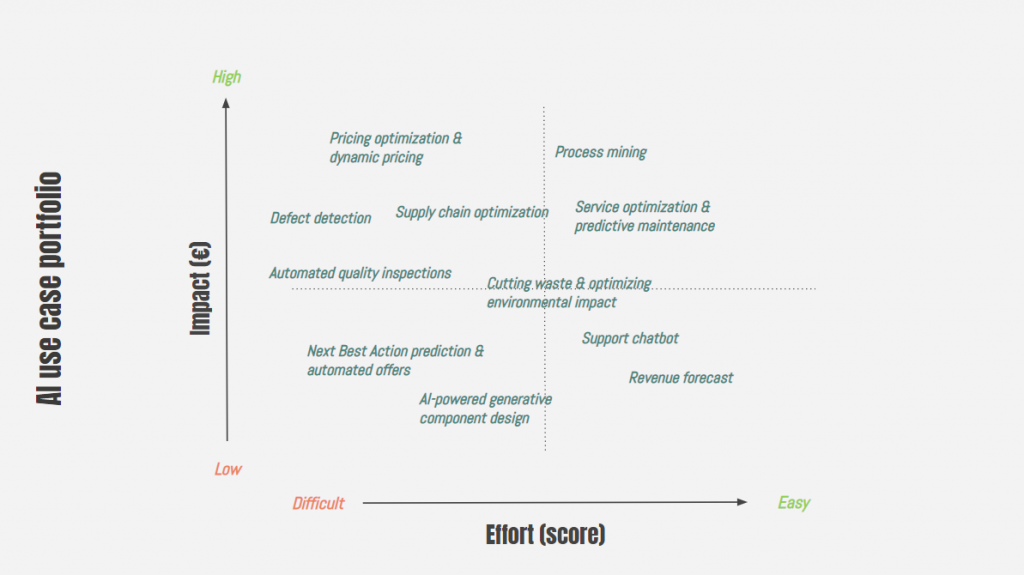
Take the data maturity quiz – know where you stand
Such a large proportion of AI projects fail due to a lack of data maturity. With proper visibility on why an organisation lacks data maturity, businesses can take active steps to fill in the gaps. Proper data maturity underpins the feasibility and impact of a use case portfolio, and ultimately, leads to successful AI projects.
In order to help organisations identify these gaps, Enterprise AI developed this data maturity quiz. With these quick and clear questions, you can identify within which of the four data maturity dimensions your business is lacking. This information is presented in an easy-to-interpret compass visualisation, so you can take tailored but decisive action. This quiz also helps you describe your data maturity journey, so you can plan and control your progress and the business impact at each stage.
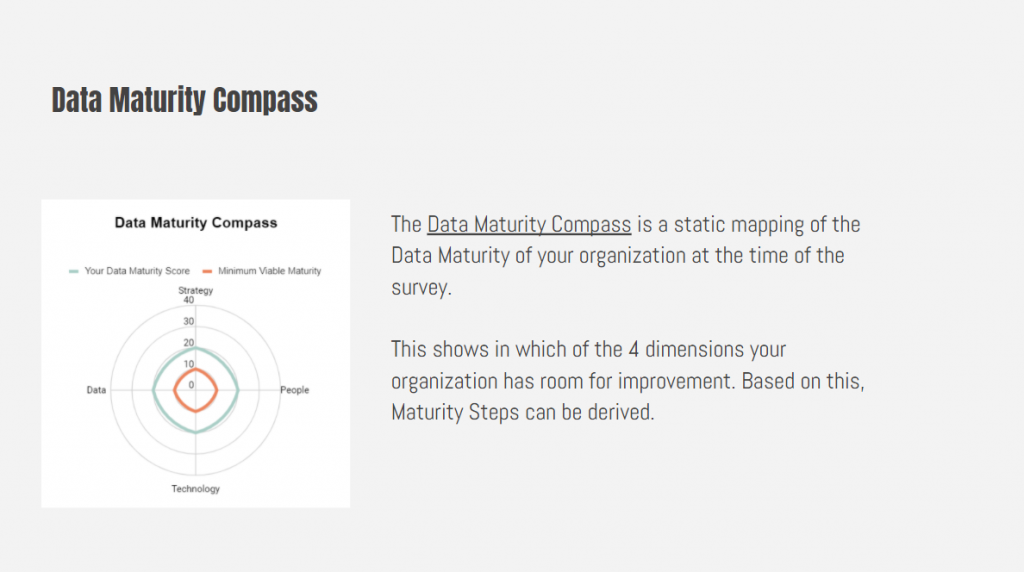
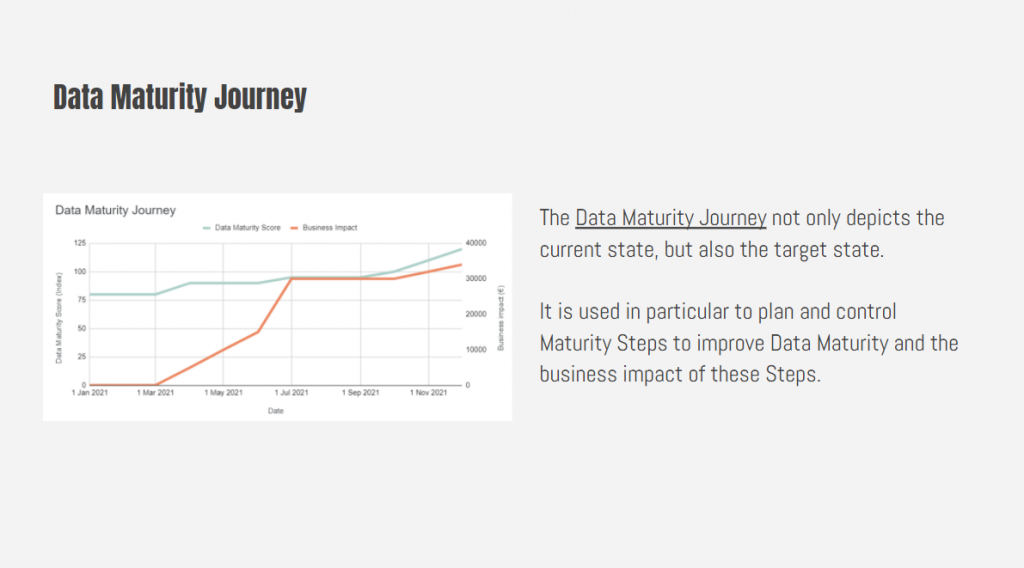
Or, to quicken the pace of your journey towards effective AI projects, Outvise offers a wealth of knowledge and expertise. With an extensive portfolio of AI and data experts from all over the world, you can match a specialised freelance data scientist with your project in as little as 48 hours. Click here to explore the platform and identify a certified data scientist today.
Our data expert Denys Holovatyi has developed a model for enterprise Data Maturity that he'll present in this article. In its earlier versions, it has been presented at the DeveloperWeek Europe 2021 conference and used as a part of data science workshops with Headfound GmbH, the Hyper Island digital school, and the University of Bundeswehr.
Denys is a data scientist & founder of EnterpriseAI Consulting, a consultancy for data science & process mining with 30+ projects in claims management, order management, customer service, marketing, and others. He also manages an AI community with 2000 members, speaks at webinars and conferences, and plays guitar in his free time.
No comments yet
There are no comments on this post yet.