Within production engineering, maximising process performance is essential to remaining competitive in fast-moving markets. This is because it enables quick decision-making while keeping costs down, which is the keystone to a successful business model. Many mathematical and digital techniques have been applied in the past, but one of the most accurate and innovative is digital twin software. With digital twin applications, engineers can simulate scenarios and fine-tune production assets with incredible precision.
Production assets are dynamic parts of the system whose operational condition will change over time. Their dynamism means that their behaviour, reliability, and functioning won’t remain constant; instead, design changes, maintenance, re-stimulation and the impact of surrounding components will affect their operation.
Certainly, human surveillance can identify and diagnose abnormalities. Sensors can improve this process; however, the fact is, “normal” doesn’t imply “optimal”. To truly optimise production, the asset needs to be operated at the proper set points. For dynamic assets, these set-points will shift over time. But how can you monitor a moving target? This is where digital twin applications come in.
Table of Contents
Digital twin software in a production process context
The Industrial Internet Consortium defines a digital twin as a digital representation of a production asset, process, or system. This digital representation captures the features and behaviour of a given entity that are appropriate to the communication, storage and analysis of certain data. As a result, the digital twin is an interface to the entity’s lifecycle. This information will include physics- and analytics-based models, time-series data, transactional data, master data and computations.
These data and visualisations can be used to get a picture of an asset’s optimal operational state. For dynamic assets or systems, optimal operations are, as mentioned, a moving target. In these scenarios, engineers can connect a live data feed to the associated devices to develop a dynamic set-point mechanism.
There are various different types of digital twin applications appropriate to different applications. These include discrete, composite, hierarchical, associational, and peer-to-peer models.
Types of digital twins
- Discrete: Twins appropriate to a single entity that requires no further deconstruction, like a gearbox or ball mill in mining. These assets can be monitored and reported on at a single level.
- Composite: An amalgam of discrete digital twins for an entity with multiple parts or components. For instance, a composite digital twin would be suitable for a production cell. In turn, the entire plant system could be made up of several composite digital twins.
- Hierarchical: Digital twins can be assembled in a hierarchy according to size or complexity. For instance, several component digital twins can comprise an equipment digital twin, equipment digital twins will comprise a production line digital twin, and so on.
- Associational: Two digital twins can be associated with each other to make a whole. For example, an oil pipeline could be associated with its production and consumption equipment digital twins.
- Peer-to-peer: A group of equipment of the same or similar types where the total effect of all the components is the simple sum of the effect of each. This model is best demonstrated with an example; in a wind farm, the engines of each turbine will form one composite digital twin.
Building a digital twin for engineering production assets
As introduced, production assets are dynamic entities. As they change their operating conditions as time goes on, so the goalposts in regard to optimisation are always moving. Digital twin is a solution to overcoming these challenges. The implementation of a digital twin with dynamic set-point optimisation on an IIoT production line requires substantial expertise in data, machine learning, and programming languages.
However, before even thinking about the design of the twin itself, the project needs to be approached from a value perspective. This means outlining a digital twin strategy to maximise the investment. A proper strategy sets up an organisation for success in the long term. Meanwhile, shooting in the dark will only lead to potentially suboptimal results for what can be a costly endeavour.
This begins by identifying a starting point and which applications should be prioritised. The team should define all potential use cases across the enterprise and rank them by which would drive the most business value. Naturally, those that rank the highest should be taken further. Next, to ensure the use cases’ viability, it is necessary to conduct an internal audit to identify the relevant data; only then can the team work towards creating an initial digital twin. The steps to creating the digital twin will comprise:
- Data processing: Collection, review, cleaning, transformation of data and cloud database maintenance.
- Simulation: Field data simulation created by automated processes in the cloud database.
- Inverse modelling: Gathering information on the model and its parameters from (historical) measurements of the subject. This includes:
- Connecting historical real-world IIoT data with simulations to identify system unknowns
- Evaluate how closely the digital twin mimics the real-world asset from historical data.
- Calibrate the digital twin software using insights from the previous steps to account for uncertainty.
- Model recommendation: Deploy automated recommendations for set-point adjustments with updates based on dynamic trends.
Later, the project can be scaled to drive enterprise-wide transformation. Digital twin applications provide a coherent strategy to effectively manage information, mitigate productivity bottlenecks, increase market agility and drive business value in the context of Industry 4.0. The opportunity digital twin mapping provides is enormous: it enables businesses to capture value with data and drive digital transformation throughout the enterprise. Let’s look closer at the specific benefits.
What challenges does a digital twin solve?
A digital twin collects data for every entity in the asset. This information is then centralised and made available to different departments for various applications. Via Application Programming Interfaces (APIs), employees can analyse the data and use it to enhance decision-making. In turn, different departments can use this shared understanding to better maintain the plant, reducing the overall lifecycle cost of operating the facility.
This is another key advantage: digital twins ease collaboration across the organisation. Digital twins can be made available to anyone, anywhere at any time, allowing for around the clock rapid reaction. With visibility for every lifecycle phase, digital twin software reduces the time spent locating, uploading and downloading information for each task. This, in turn, reduces the amount of costly downtime that maintenance would otherwise cause.
Moreover, digital twin software can go beyond optimisation and provide the basis for innovation. It can underpin the development of AI applications to consume and enrich data generated by the digital twin. Or, it can be used to develop high-fidelity virtual sensors using physics-based models. These can then be incorporated into the digital twin to serve as a proxy for physical measurements.
Digital twin case study for aerospace engineering
To demonstrate the value of a digital twin in production engineering, we’ll conclude with a brief case study. A commercial aircraft manufacturer requires various parts from different suppliers: engines, landing gear, avionics, and so on. A digital twin of an aircraft can be created via a composite of digital twins of these constituent parts. These twins should be interoperable and maintained for as-operated and as-maintained status. This will ensure greater precision along the production line, faster bottleneck identification, and ultimately, a more reliable finished product.
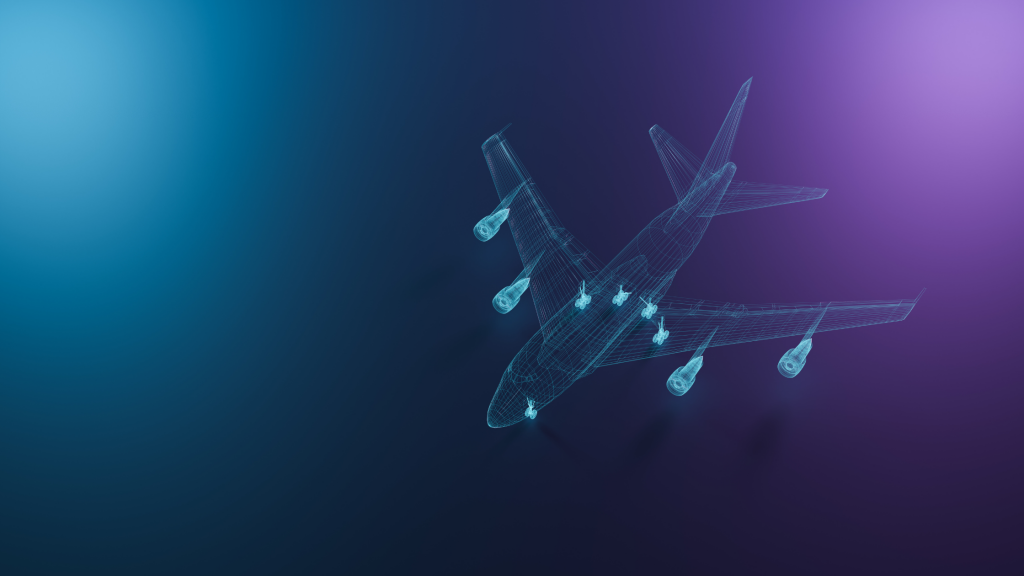
The digital twin of this finished product, the aircraft, will help with predictive maintenance, operating efficiencies like fuel consumption, and the formulation of asset maintenance strategies. This can provide substantial benefits for the customer and manufacturer as, considering that the lifetime of an aircraft is often several decades, the total cost of maintenance can exceed the original cost of the aircraft. With digital twin software, companies can identify areas for predictive maintenance and slim down the cost of upkeep as much as possible.
Identify the expertise you need to kick-start a digital twin strategy
In summary, a properly planned digital twin strategy can have myriad benefits for companies in the manufacturing and engineering sector. These digital simulations of plants, products, and their constituent parts can refine processes, enhance collaboration and save on downtime. This, in turn, boosts production, saves money and strengthens the business. As demonstrated in the brief case study above, these benefits can also be passed on to the customer.
However, as touched on, developing an effective digital twin is an endeavour that requires careful planning and expertise. This expertise will vary depending on the digital twin’s application and the associated sector, so you need a professional with the relevant expertise. And, for project-based work, how can you hire this individual in the most cost-effective way possible?
The answer lies in the freelance market. There are thousands of highly experienced professionals providing consultancy and development for such projects. All you have to do is find them – and this is where Outvise comes in. Outvise’s curated portfolio of digital experts comprises digital twin experts, data scientists and engineers, IoT experts, and many more profiles to kick-start your enterprise’s digital twin strategy. Plus, thanks to the unique project-matching algorithm, you can find an expert with experience relevant to your sector in as little as 48 hours. Explore the platform here.
Michael is the Technical Lead at Cedar Analytics IoT Services, an Industrial Internet of Things company offering consultancy and tools- hardware and connectivity to businesses. He is a passionate technology professional with close to a decade experience.
He is a result driven individual with a passion for emerging technologies developing businesses, and helping them grow. He is also a skilled and experienced problem solver who optimizes business profitability by leveraging technology.
No comments yet
There are no comments on this post yet.