“Artificial intelligence is whatever hasn’t been done yet”
– Larry Tesler, early 1970
Last year, artificial intelligence (AI) hit the big time. The storm started in 2022 when tools such as ChatGPT, Stable Diffusion and Midjourney were launched. These are examples of generative artificial intelligence solutions, each with its unique features and applications. ChatGPT, for instance, is a conversational AI model developed by OpenAI, designed to generate text responses based on prompts. The human-like tone of the tool has prompted anxiety in popular media, unleashing a host of AI myths and doomsday tales.
Despite the apprehension in some circles, the adoption rate of these AI tools exploded shortly after their launch, driven by their versatility and potential across various industries. Particularly noteworthy is the aforementioned Large Language Model (LLM) ChatGPT, which surpassed 1 million users just 5 days after its release. Since then, its user base has skyrocketed to over 180 million users, solidifying its position as one of the most widely used AI tools. As of the time of writing, ChatGPT continues to evolve, shaping the landscape of human-computer interaction.
While the rapid advancements in artificial intelligence have led to groundbreaking innovations and widespread adoption, they have also given rise to numerous misconceptions and myths. Understanding the reality behind these AI myths is crucial in deploying AI strategically and effectively. In this article, I’ll debunk some of the most common AI myths, shedding light on the truth behind the technology and its implications for society.
Table of Contents
A potted history of AI hysteria
The proliferation of generative AI has undeniably sparked concerns, with some making dire predictions asserting that it’s only a matter of time before AI replaces humans. But is this vision rooted in reality, or are we succumbing to unfounded fears? To divorce the AI myths from reality, history offers us a poignant perspective; especially considering that when you unpack the subject, AI isn’t all that new.
Cast your mind back to 1997 when IBM’s supercomputer Deep Blue made headlines by defeating world chess champion Garry Kasparov in a series of matches. The event sent shockwaves through the world, with many heralding it as the demise of human chess mastery. Deep Blue’s victory was seen as a watershed moment, a triumph of machine over human intellect.
Yet, as time went on, it became clear that this victory was not a harbinger of human obsolescence. Chess enthusiasts continued to refine their strategies and human players maintained their dominance in the game, demonstrating that while AI could excel in specific tasks, it still lacked the nuanced understanding and creative insight inherent in human thought.
Similarly, in 2011, IBM’s Watson captured the public imagination by outwitting former champions on the TV quiz show Jeopardy. The victory was celebrated as a triumph of AI over human intellect, with many speculating that Watson’s capabilities would revolutionise fields such as medicine. However, despite the initial hype, Watson’s impact on medicine fell short of the lofty predictions. While AI technologies have certainly made inroads in healthcare, the complexity of medical decision-making and the intricacies of human biology have proven to be formidable challenges for AI systems.
Then, in 2017, came the triumph of Google’s DeepMind AlphaGo over the world’s number one Go player, Ke Jie. The ancient game of Go, with its complexity and depth, had long been considered a bastion of human intelligence. AlphaGo’s victory seemed to signify a new era, where AI surpassed human expertise in yet another domain.
However, just as with Deep Blue and Watson, AlphaGo’s success did not spell the end of human involvement in Go. Instead, it served as a reminder of the power of collaboration between humans and AI. While AI could indeed surpass human capabilities in certain narrow tasks, the essence of human ingenuity and creativity remained unparalleled.
Is AI a danger to humanity?
Despite these recent encounters with AI myths and their realities, doomsday narratives persist. Some experts and pundits, such as Geoffrey Hinton, a recipient of the 2018 Turing Award alongside Yoshua Bengio and Yann LeCun, are sounding the alarm. As one of the pioneers of deep learning, Hinton has repeatedly expressed concerns about the trajectory of AI advancement, suggesting the possibility of machines surpassing human control. He co-authored the paper “Managing AI Risks in an Era of Rapid Progress,” which highlights potential risks associated with advanced AI systems, including large-scale social harm and loss of human control over autonomous AI while proposing urgent priorities for AI research and governance.
On the opposing side, many specialists acknowledge the profound impact of generative AI while also asserting that AI is far from posing an existential threat to humanity. Rodney Brooks, former Director of the Computer Science and Artificial Intelligence Laboratory at MIT, exemplifies this perspective. In an interview, Brooks celebrates the progress made in AI but also emphasises its inherent limitations. A classic question is “Can AI become self-aware?” Brooks contends that we are nowhere near achieving the elusive goal of artificial general intelligence (AGI) or self-aware AI systems. For now, it seems unlikely that ChatGPT will take over the world.
Indeed, in light of the question, “Is ChatGPT a threat to humanity?” the truth likely lies somewhere between these two extremes. While the current wave of AI presents significant opportunities, it also demands careful attention to ensure that its positive aspects are maximised while mitigating the possibility that certain AI myths become reality. One such issue is bias and discrimination; as such, ethics in AI emerges as a crucial topic for the coming years.
Bias and discrimination are particularly important themes as AI systems are trained on large datasets. if these datasets contain biased or incomplete information, the AI models may perpetuate or even exacerbate existing biases. For example, facial recognition systems have been found to exhibit racial and gender biases, leading to discriminatory outcomes. Addressing bias in AI requires careful data collection, algorithm design, and ongoing monitoring to ensure fairness and equity.
Furthermore, there are broader societal implications of AI, including its impact on employment, inequality and autonomy. As AI technologies automate tasks and reshape industries, there is a risk of job displacement and economic disruption. Ensuring a just transition for workers and mitigating the widening gap between the technologically skilled and the economically disadvantaged are pressing challenges. Addressing these ethical considerations requires interdisciplinary collaboration, stakeholder engagement and a commitment to ethical AI principles.
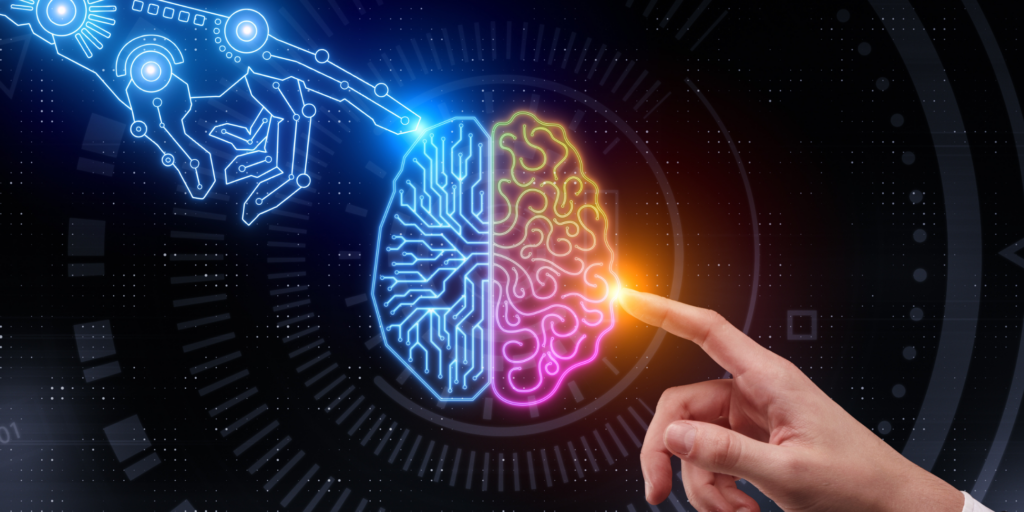
Normalisation and navigation
Nevertheless, it’s important to remember that as Larry Tesler once said, “Artificial intelligence is whatever hasn’t been done yet”. The reality is, numerous disruptive AI algorithms have seamlessly integrated into our daily lives without major disruptions. Consider, for instance, the ubiquitous presence of Google Maps, which has rendered paper maps virtually obsolete. As we navigate the future and confront these AI myths and their subsequent realities, much yet is to be determined.
Indeed, not only is there the likelihood that AI usage becomes increasingly normalised, but that progress in the field isn’t necessarily an exponential curve. Despite the tremendous progress in AI research and development, significant challenges and limitations remain. For instance, AI technologies face technical limitations and constraints, such as data scarcity, computational complexity and domain specificity. AI models trained on limited or biased data may generalise poorly or exhibit limited performance in real-world scenarios.
Scaling AI algorithms to handle large datasets or complex tasks can also strain computational resources and infrastructure. Overcoming these technical challenges requires advancements in machine learning algorithms, data collection techniques and computing hardware. Equally, there are legal and regulatory challenges associated with AI deployment. If authorities engage with the aforementioned ethical challenges, navigating the complex landscape of AI governance, intellectual property rights, liability, and accountability could prove challenging for developers.
Certainly, those in the field shouldn’t blindly occupy themselves with the task of infinite progress. Ensuring that AI technologies are developed and deployed responsibly, ethically and equitably is essential for building trust, fostering innovation, and maximising the societal benefits of AI.
If AI isn’t taking over the world, how will it affect business?
The ongoing discourse surrounding the potential and risks of AI within society is undeniably captivating, permeating discussions in nearly every company and organisation. Questions like “How will AI impact my business?” or “How can AI enhance my company’s efficiency?” have become commonplace. While these inquiries hold merit, I advocate for a nuanced approach. Similar to the so-called “big data wave” of 2014, the current “AI wave” may prove disappointing for organisations that fail to adopt a value or use-case-oriented strategy.
What exactly do I mean by a use-case approach? Rather than adopting a blanket “let’s use AI for our company” stance, I contend that it’s crucial to first identify the primary business challenges facing a company and assess whether leveraging data can address them. Questions such as “How can I boost sales?” or “How can I streamline operational costs?” must be clearly defined before delving into the realm of AI implementation. Without concrete definitions or reliable data to support your answers, grappling with the AI question is futile. It’s an inconvenient truth that a significant percentage of companies are ill-prepared for this undertaking.
Assuming these questions are well-defined and backed by robust data – note that quality and reliability trump sheer volume in data importance – the focus should shift to determining the most effective data approach to tackle the business challenge at hand. For example, in the transportation industry, where transportation costs often constitute a significant expense, optimising travel time can lead to substantial cost reductions. Does this necessitate AI? Not necessarily, unless one considers a traditional “route optimisation” algorithm as an AI endeavour – a claim that some may make, albeit erroneously, to capitalise on AI hype.
The prevalence of low-hanging fruit within companies underscores the importance of identifying and addressing one’s own “route optimization use case,” which can yield tangible benefits without the need for generative AI. While genuine generative AI solutions have garnered rapid adoption due to their efficacy in solving real problems, ranging from image generation to wildfire detection, it’s essential to scrutinize their relevance to specific business needs. Indeed, the allure of AI can sometimes overshadow simpler, more straightforward solutions.
Sort the AI myths from the real opportunities
The key lies in pinpointing the problems you aim to solve. While AI solutions undoubtedly hold promise, it’s essential to weigh the option of building from scratch against integrating default solutions already embedded in everyday tools. As always, the “make or buy” question remains pertinent, necessitating careful consideration before committing resources to extensive internal developments.
Ultimately, the success of AI implementation hinges on a holistic understanding of organisational needs, coupled with a discerning approach to selecting and deploying the most suitable solutions. By reframing the discourse surrounding AI from one of hype and speculation to one grounded in practicality and purpose, companies can navigate the AI landscape with greater confidence and clarity, maximising its potential benefits while mitigating potential pitfalls.
It’s worth acknowledging the prevalence of companies leveraging AI as mere clickbait to attract capital. AI brings amazing opportunities that every company should explore, but it becomes crucial not to be fooled by press releases and separate the AI myths from reality. To take the first steps in unpicking this conundrum, look into getting an expert on board. Dive into Outvise’s pool of seasoned AI Specialists and Data Analysts and get the people you need to harness the immense technological potential that lies within our grasp.
VP Data Analytics at Holaluz.
With over 20 years of experience in digital projects, Manuel has a passion for storytelling through data. As a seasoned professional, he has successfully led and managed teams specialising in Analytics, Insights, and Data Science. Manuel believes in infusing technology with humanism to create meaningful and impactful solutions for the digital age.
No comments yet
There are no comments on this post yet.